

We establish a framework for releasing certain kinds of peer-review data while preserving privacy of reviewer assignments. There is indeed a challenge in releasing any data publicly due to requirements on the anonymity of reviewers for each paper. Privacy: A major impediment towards research on peer review is the dearth of data.We address this issue by exploiting primacy effects to design an algorithm that balances (i) amount of skew in the bids, and (ii) reviewer satisfaction.

Such bidding is known to be highly skewed with a large number of papers getting zero or insufficient bids.
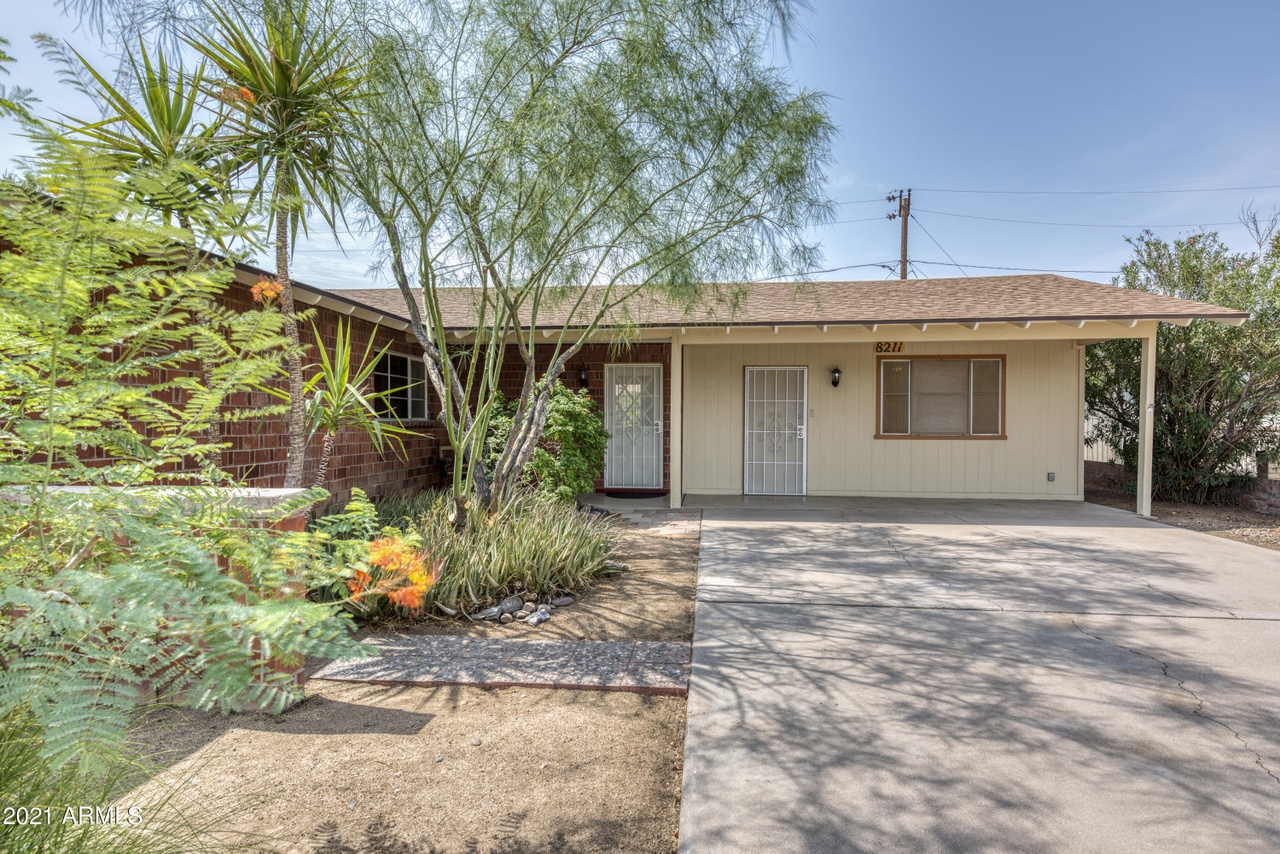
They try to get assigned, and then accept each other's papers. Malicious coalitions: It has recently been discovered that in paper and grant peer review, some people form malicious coalitions.We also design a statistical test to check for large-scale strategic behavior ( link). We evaluate the feasibility and efficacy of strategyproofing via empirical evaluations ( link) as well as theoretically ( link). Lone-wolf strategic review: In competitive peer review, reviewers can increase chances of their own papers getting accepted by manipulating the reviews they provide.We also provide an empirical analysis on IJCAI 2017 ( link). We prove that surprisingly, this is the only method which meets three natural requirements. We develop a novel method to mitigate such subjectivity by ensuring that every paper is judged by the same yardstick. Subjectivity: It is common to see a handful of reviewers reject a highly novel paper, because they view extensive experiments more important than novelty, whereas the community as a whole would have embraced the novelty.This algorithm was used in ICML 2020 and performed very well on common metrics of evaluation ( link). Simultaneously, the algorithm guarantees statistical accuracy of the review procedure. We design an algorithm to assign reviewers to papers which guarantees fairness of assignment to all papers. Reviewer assignment: Commonly employed algorithms for assigning reviewers to papers can be unfair to certain papers (e.g., to interdisciplinary or novel papers).This also leads to a surprising insight into the eternal debate between ratings and rankings ( link). If these biases are a priori unknown, how would can calibrate the reviewers (from say, just one review obtained per reviewer)? We design a novel randomized estimator that can handle arbitrary and even adversarial miscalibrations. Miscalibration: Reviewers are often miscalibrated: some reviewer may be lenient and always provide scores greater than 5/10, while some other may be strict and never provide more than 5/10.We also design algorithms to test for biases in review text rather than just review scores ( link).
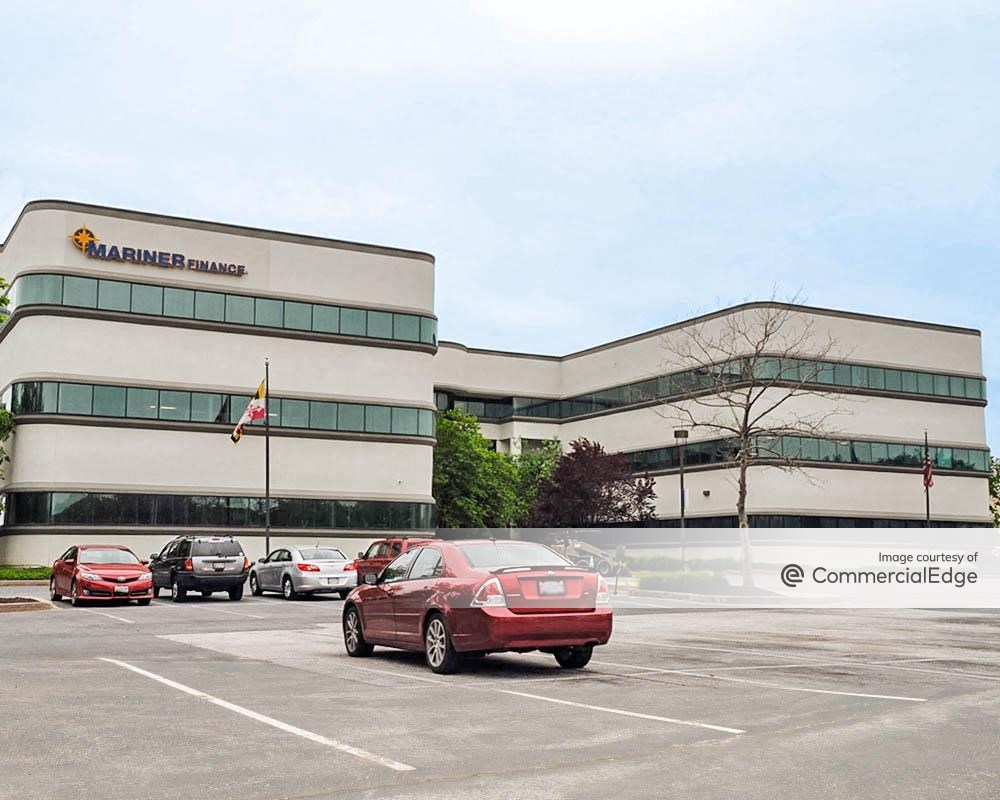
double blind reviewing, with a FAQ "Where is the evidence of bias in reviewing in my community?'' We design statistical tests for biases in peer review, that accommodates the various idiosyncrasies of the peer review process.
